Radiomics to Boost SCLC Detection
By Rachel Ernst |
Small-cell lung cancer (SCLC) represents a low percentage of lung cancers. However, this type tends to cause damage quicker as it spreads at a faster rate than other cancers. Advancements in radiomics can help detect SCLC more efficiently.
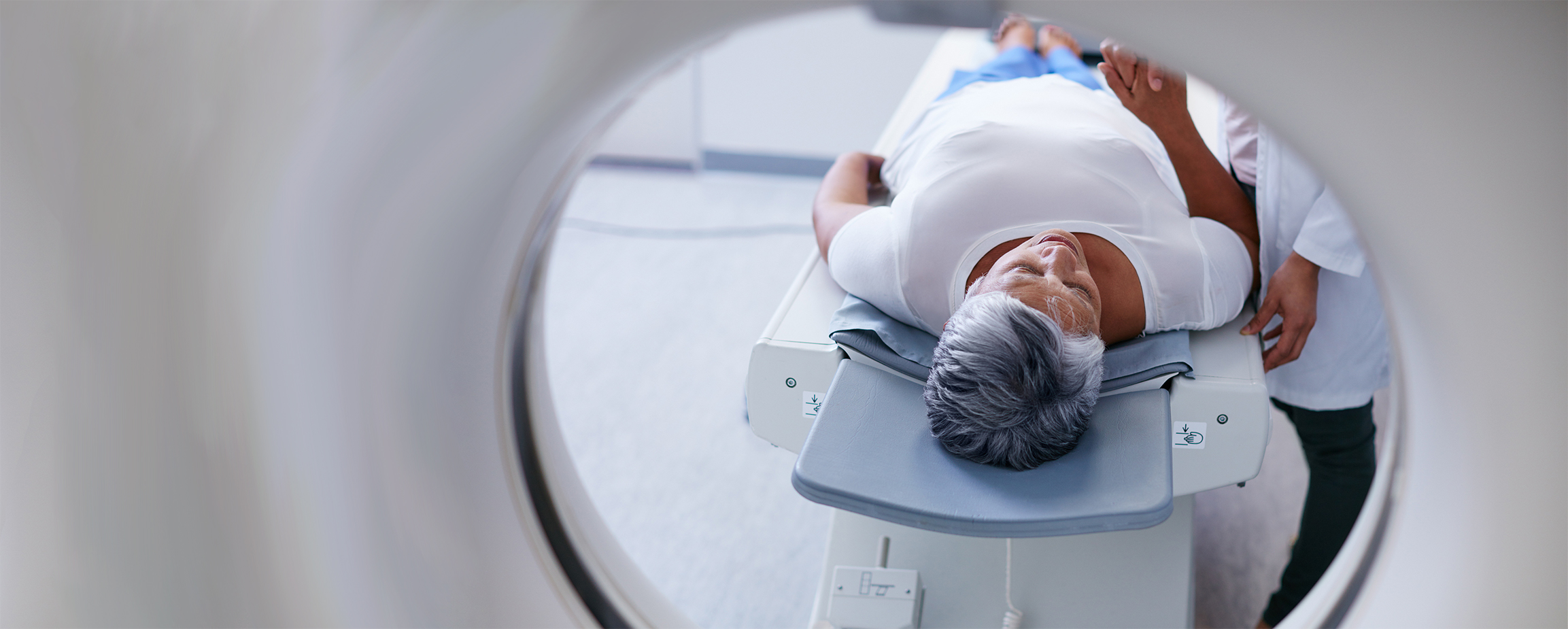
Study Finds Radiomics Can Help Treat Lung Cancer
Researchers at the Center for Computational Imaging and Personalized Diagnostics (CCIPD) at Case Western Reserve University have used artificial intelligence (AI) to identify a set of patterns using radiomics. Using imaging tests like CT scans, the technology can predict a cancer patient’s response to chemotherapy. The radionic patterns may play a significant role in providing early detection of small cell lung cancer and improving survival rates.
Before chemotherapy treatment, researchers collect data containing a set of patterns. AI has been used to predict treatment outcomes for non-small cell lung cancer and other forms, but rarely small-cell lung cancer. Small-cell lung cancer (SCLC) only represents about 13% of all lung cancers but spreads and grows at faster rates.
Researchers hope the radiomic scans can help improve treatment response for SCLC, which has always been challenging. Long-term outcomes of AI-derived images will help researchers examine the success of the technique. The radiomics initiative is currently taking place at three Boston treatment centers: Harvard Medical School, Dana-Farber Cancer Institute, and Brigham and Women’s Hospital.
Earlier this year, researchers in Cleveland, Ohio, released results from a novel study of non-invasive radiomic signature on CT scans in the use of chemotherapy treatment prediction. The study found the technology can predict a patient’s response to platinum-based chemotherapy and their prognosis. Both predictions can improve the overall survival of patients with SCLC.
Future Applications Could Be Significant
In 2012, Philippe Lambin defined radiomics as “the high-throughput mining of quantitative image features from standard-of-care medical imaging.” Lambin believed that radiomics could enable data to be extracted and applied within clinical decision support systems.
Back then, radiomic research began to explore the possibility of improving diagnostic, prognostic, and predictive accuracy in cancer research. The concept of applying algorithms to the datasets collected from CT, MRI, PET, and ultrasound imaging may be the key to future diagnostic procedures. With the radiomic information, researchers can predict tumor stage, tumor metabolism, overall patient survival, metastasis, treatment response, and underlying gene expression profiles.
While conventional treatment options such as chemotherapy remain the standard, the potential for future radiomics applications could be significant. In the clinical setting, researchers predict the use of AI to detect and treat lung cancer could become commonplace across the world.
Radiomics May Detect Breast, Lung, and Colon Cancers
Studies over the last decade focused on using radiomic analysis with CT or PET scans. Using statistical modeling or a machine-learning approach, the data combined with the scans could predict EGFR mutations.
Recently, however, deep learning approaches use the data after treatment to establish prognostic markers. In essence, the rapidly developing approach may improve the prognosis of patients with various types of cancer, including breast and colon cancers.
Some researchers believe they have enough scientific evidence of success using radiomic features. More research is needed in regard to radiomic biomarkers to improve diagnostic and predictive power. Other issues of image quality and the requirement of large datasets make it complicated. Overall, radiomics is a relatively cheap approach to precision medicine.